浅谈Python实现Apriori算法介绍
导读:
随着大数据概念的火热,啤酒与尿布的故事广为人知。我们如何发现买啤酒的人往往也会买尿布这一规律?数据挖掘中的用于挖掘频繁项集和关联规则的Apriori算法可以告诉我们。本文首先对Apriori算法进行简介,而后进一步介绍相关的基本概念,之后详细的介绍Apriori算法的具体策略和步骤,最后给出Python实现代码。
1.Apriori算法简介
Apriori算法是经典的挖掘频繁项集和关联规则的数据挖掘算法。A priori在拉丁语中指"来自以前"。当定义问题时,通常会使用先验知识或者假设,这被称作"一个先验"(a priori)。Apriori算法的名字正是基于这样的事实:算法使用频繁项集性质的先验性质,即频繁项集的所有非空子集也一定是频繁的。Apriori算法使用一种称为逐层搜索的迭代方法,其中k项集用于探索(k+1)项集。首先,通过扫描数据库,累计每个项的计数,并收集满足最小支持度的项,找出频繁1项集的集合。该集合记为L1。然后,使用L1找出频繁2项集的集合L2,使用L2找出L3,如此下去,直到不能再找到频繁k项集。每找出一个Lk需要一次数据库的完整扫描。Apriori算法使用频繁项集的先验性质来压缩搜索空间。
2. 基本概念
- 项与项集:设itemset={item1, item_2, …, item_m}是所有项的集合,其中,item_k(k=1,2,…,m)成为项。项的集合称为项集(itemset),包含k个项的项集称为k项集(k-itemset)。
- 事务与事务集:一个事务T是一个项集,它是itemset的一个子集,每个事务均与一个唯一标识符Tid相联系。不同的事务一起组成了事务集D,它构成了关联规则发现的事务数据库。
- 关联规则:关联规则是形如A=>B的蕴涵式,其中A、B均为itemset的子集且均不为空集,而A交B为空。
- 支持度(support):关联规则的支持度定义如下:
其中表示事务包含集合A和B的并(即包含A和B中的每个项)的概率。注意与P(A or B)区别,后者表示事务包含A或B的概率。
置信度(confidence):关联规则的置信度定义如下:
项集的出现频度(support count):包含项集的事务数,简称为项集的频度、支持度计数或计数。
频繁项集(frequent itemset):如果项集I的相对支持度满足事先定义好的最小支持度阈值(即I的出现频度大于相应的最小出现频度(支持度计数)阈值),则I是频繁项集。
强关联规则:满足最小支持度和最小置信度的关联规则,即待挖掘的关联规则。
3. 实现步骤
一般而言,关联规则的挖掘是一个两步的过程:
- 找出所有的频繁项集
- 由频繁项集产生强关联规则
3.1挖掘频繁项集
3.1.1相关定义
连接步骤:频繁(k-1)项集Lk-1的自身连接产生候选k项集Ck
Apriori算法假定项集中的项按照字典序排序。如果Lk-1中某两个的元素(项集)itemset1和itemset2的前(k-2)个项是相同的,则称itemset1和itemset2是可连接的。所以itemset1与itemset2连接产生的结果项集是{itemset1[1], itemset1[2], …, itemset1[k-1], itemset2[k-1]}。连接步骤包含在下文代码中的create_Ck函数中。
剪枝策略
由于存在先验性质:任何非频繁的(k-1)项集都不是频繁k项集的子集。因此,如果一个候选k项集Ck的(k-1)项子集不在Lk-1中,则该候选也不可能是频繁的,从而可以从Ck中删除,获得压缩后的Ck。下文代码中的is_apriori函数用于判断是否满足先验性质,create_Ck函数中包含剪枝步骤,即若不满足先验性质,剪枝。
删除策略
基于压缩后的Ck,扫描所有事务,对Ck中的每个项进行计数,然后删除不满足最小支持度的项,从而获得频繁k项集。删除策略包含在下文代码中的generate_Lk_by_Ck函数中。
3.1.2 步骤
- 每个项都是候选1项集的集合C1的成员。算法扫描所有的事务,获得每个项,生成C1(见下文代码中的create_C1函数)。然后对每个项进行计数。然后根据最小支持度从C1中删除不满足的项,从而获得频繁1项集L1。
- 对L1的自身连接生成的集合执行剪枝策略产生候选2项集的集合C2,然后,扫描所有事务,对C2中每个项进行计数。同样的,根据最小支持度从C2中删除不满足的项,从而获得频繁2项集L2。
- 对L2的自身连接生成的集合执行剪枝策略产生候选3项集的集合C3,然后,扫描所有事务,对C3每个项进行计数。同样的,根据最小支持度从C3中删除不满足的项,从而获得频繁3项集L3。
- 以此类推,对Lk-1的自身连接生成的集合执行剪枝策略产生候选k项集Ck,然后,扫描所有事务,对Ck中的每个项进行计数。然后根据最小支持度从Ck中删除不满足的项,从而获得频繁k项集。
3.2 由频繁项集产生关联规则
一旦找出了频繁项集,就可以直接由它们产生强关联规则。产生步骤如下:
对于每个频繁项集itemset,产生itemset的所有非空子集(这些非空子集一定是频繁项集);
对于itemset的每个非空子集s,如果,则输出
,其中min_conf是最小置信度阈值。
4. 样例以及Python实现代码
下图是《数据挖掘:概念与技术》(第三版)中挖掘频繁项集的样例图解。
本文基于该样例的数据编写Python代码实现Apriori算法。代码需要注意如下两点:
- 由于Apriori算法假定项集中的项是按字典序排序的,而集合本身是无序的,所以我们在必要时需要进行set和list的转换;
- 由于要使用字典(support_data)记录项集的支持度,需要用项集作为key,而可变集合无法作为字典的key,因此在合适时机应将项集转为固定集合frozenset。
""" # Python 2.7 # Filename: apriori.py # Author: llhthinker # Email: hangliu56[AT]gmail[DOT]com # Blog: http://www.cnblogs.com/llhthinker/p/6719779.html # Date: 2017-04-16 """ def load_data_set(): """ Load a sample data set (From Data Mining: Concepts and Techniques, 3th Edition) Returns: A data set: A list of transactions. Each transaction contains several items. """ data_set = [['l1', 'l2', 'l5'], ['l2', 'l4'], ['l2', 'l3'], ['l1', 'l2', 'l4'], ['l1', 'l3'], ['l2', 'l3'], ['l1', 'l3'], ['l1', 'l2', 'l3', 'l5'], ['l1', 'l2', 'l3']] return data_set def create_C1(data_set): """ Create frequent candidate 1-itemset C1 by scaning data set. Args: data_set: A list of transactions. Each transaction contains several items. Returns: C1: A set which contains all frequent candidate 1-itemsets """ C1 = set() for t in data_set: for item in t: item_set = frozenset([item]) C1.add(item_set) return C1 def is_apriori(Ck_item, Lksub1): """ Judge whether a frequent candidate k-itemset satisfy Apriori property. Args: Ck_item: a frequent candidate k-itemset in Ck which contains all frequent candidate k-itemsets. Lksub1: Lk-1, a set which contains all frequent candidate (k-1)-itemsets. Returns: True: satisfying Apriori property. False: Not satisfying Apriori property. """ for item in Ck_item: sub_Ck = Ck_item - frozenset([item]) if sub_Ck not in Lksub1: return False return True def create_Ck(Lksub1, k): """ Create Ck, a set which contains all all frequent candidate k-itemsets by Lk-1's own connection operation. Args: Lksub1: Lk-1, a set which contains all frequent candidate (k-1)-itemsets. k: the item number of a frequent itemset. Return: Ck: a set which contains all all frequent candidate k-itemsets. """ Ck = set() len_Lksub1 = len(Lksub1) list_Lksub1 = list(Lksub1) for i in range(len_Lksub1): for j in range(1, len_Lksub1): l1 = list(list_Lksub1[i]) l2 = list(list_Lksub1[j]) l1.sort() l2.sort() if l1[0:k-2] == l2[0:k-2]: Ck_item = list_Lksub1[i] | list_Lksub1[j] # pruning if is_apriori(Ck_item, Lksub1): Ck.add(Ck_item) return Ck def generate_Lk_by_Ck(data_set, Ck, min_support, support_data): """ Generate Lk by executing a delete policy from Ck. Args: data_set: A list of transactions. Each transaction contains several items. Ck: A set which contains all all frequent candidate k-itemsets. min_support: The minimum support. support_data: A dictionary. The key is frequent itemset and the value is support. Returns: Lk: A set which contains all all frequent k-itemsets. """ Lk = set() item_count = {} for t in data_set: for item in Ck: if item.issubset(t): if item not in item_count: item_count[item] = 1 else: item_count[item] += 1 t_num = float(len(data_set)) for item in item_count: if (item_count[item] / t_num) >= min_support: Lk.add(item) support_data[item] = item_count[item] / t_num return Lk def generate_L(data_set, k, min_support): """ Generate all frequent itemsets. Args: data_set: A list of transactions. Each transaction contains several items. k: Maximum number of items for all frequent itemsets. min_support: The minimum support. Returns: L: The list of Lk. support_data: A dictionary. The key is frequent itemset and the value is support. """ support_data = {} C1 = create_C1(data_set) L1 = generate_Lk_by_Ck(data_set, C1, min_support, support_data) Lksub1 = L1.copy() L = [] L.append(Lksub1) for i in range(2, k+1): Ci = create_Ck(Lksub1, i) Li = generate_Lk_by_Ck(data_set, Ci, min_support, support_data) Lksub1 = Li.copy() L.append(Lksub1) return L, support_data def generate_big_rules(L, support_data, min_conf): """ Generate big rules from frequent itemsets. Args: L: The list of Lk. support_data: A dictionary. The key is frequent itemset and the value is support. min_conf: Minimal confidence. Returns: big_rule_list: A list which contains all big rules. Each big rule is represented as a 3-tuple. """ big_rule_list = [] sub_set_list = [] for i in range(0, len(L)): for freq_set in L[i]: for sub_set in sub_set_list: if sub_set.issubset(freq_set): conf = support_data[freq_set] / support_data[freq_set - sub_set] big_rule = (freq_set - sub_set, sub_set, conf) if conf >= min_conf and big_rule not in big_rule_list: # print freq_set-sub_set, " => ", sub_set, "conf: ", conf big_rule_list.append(big_rule) sub_set_list.append(freq_set) return big_rule_list if __name__ == "__main__": """ Test """ data_set = load_data_set() L, support_data = generate_L(data_set, k=3, min_support=0.2) big_rules_list = generate_big_rules(L, support_data, min_conf=0.7) for Lk in L: print "="*50 print "frequent " + str(len(list(Lk)[0])) + "-itemsets\t\tsupport" print "="*50 for freq_set in Lk: print freq_set, support_data[freq_set] print print "Big Rules" for item in big_rules_list: print item[0], "=>", item[1], "conf: ", item[2]
代码运行结果截图如下:
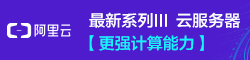
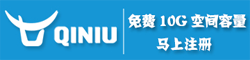