keras模型保存为tensorflow的二进制模型方式
最近需要将使用keras训练的模型移植到手机上使用, 因此需要转换到tensorflow的二进制模型。
折腾一下午,终于找到一个合适的方法,废话不多说,直接上代码:
# coding=utf-8 import sys from keras.models import load_model import tensorflow as tf import os import os.path as osp from keras import backend as K def freeze_session(session, keep_var_names=None, output_names=None, clear_devices=True): """ Freezes the state of a session into a prunned computation graph. Creates a new computation graph where variable nodes are replaced by constants taking their current value in the session. The new graph will be prunned so subgraphs that are not neccesary to compute the requested outputs are removed. @param session The TensorFlow session to be frozen. @param keep_var_names A list of variable names that should not be frozen, or None to freeze all the variables in the graph. @param output_names Names of the relevant graph outputs. @param clear_devices Remove the device directives from the graph for better portability. @return The frozen graph definition. """ from tensorflow.python.framework.graph_util import convert_variables_to_constants graph = session.graph with graph.as_default(): freeze_var_names = list(set(v.op.name for v in tf.global_variables()).difference(keep_var_names or [])) output_names = output_names or [] output_names += [v.op.name for v in tf.global_variables()] input_graph_def = graph.as_graph_def() if clear_devices: for node in input_graph_def.node: node.device = "" frozen_graph = convert_variables_to_constants(session, input_graph_def, output_names, freeze_var_names) return frozen_graph input_fld = sys.path[0] weight_file = 'your_model.h5' output_graph_name = 'tensor_model.pb' output_fld = input_fld + '/tensorflow_model/' if not os.path.isdir(output_fld): os.mkdir(output_fld) weight_file_path = osp.join(input_fld, weight_file) K.set_learning_phase(0) net_model = load_model(weight_file_path) print('input is :', net_model.input.name) print ('output is:', net_model.output.name) sess = K.get_session() frozen_graph = freeze_session(K.get_session(), output_names=[net_model.output.op.name]) from tensorflow.python.framework import graph_io graph_io.write_graph(frozen_graph, output_fld, output_graph_name, as_text=False) print('saved the constant graph (ready for inference) at: ', osp.join(output_fld, output_graph_name))
上面代码实现保存到当前目录的tensor_model目录下。
验证:
import tensorflow as tf import numpy as np import PIL.Image as Image import cv2 def recognize(jpg_path, pb_file_path): with tf.Graph().as_default(): output_graph_def = tf.GraphDef() with open(pb_file_path, "rb") as f: output_graph_def.ParseFromString(f.read()) tensors = tf.import_graph_def(output_graph_def, name="") print tensors with tf.Session() as sess: init = tf.global_variables_initializer() sess.run(init) op = sess.graph.get_operations() for m in op: print(m.values()) input_x = sess.graph.get_tensor_by_name("convolution2d_1_input:0") #具体名称看上一段代码的input.name print input_x out_softmax = sess.graph.get_tensor_by_name("activation_4/Softmax:0") #具体名称看上一段代码的output.name print out_softmax img = cv2.imread(jpg_path, 0) img_out_softmax = sess.run(out_softmax, feed_dict={input_x: 1.0 - np.array(img).reshape((-1,28, 28, 1)) / 255.0}) print "img_out_softmax:", img_out_softmax prediction_labels = np.argmax(img_out_softmax, axis=1) print "label:", prediction_labels pb_path = 'tensorflow_model/constant_graph_weights.pb' img = 'test/6/8_48.jpg' recognize(img, pb_path)
补充知识:如何将keras训练好的模型转换成tensorflow的.pb的文件并在TensorFlow serving环境调用
首先keras训练好的模型通过自带的model.save()保存下来是 .model (.h5) 格式的文件
模型载入是通过 my_model = keras . models . load_model( filepath )
要将该模型转换为.pb 格式的TensorFlow 模型,代码如下:
# -*- coding: utf-8 -*- from keras.layers.core import Activation, Dense, Flatten from keras.layers.embeddings import Embedding from keras.layers.recurrent import LSTM from keras.layers import Dropout from keras.layers.wrappers import Bidirectional from keras.models import Sequential,load_model from keras.preprocessing import sequence from sklearn.model_selection import train_test_split import collections from collections import defaultdict import jieba import numpy as np import sys reload(sys) sys.setdefaultencoding('utf-8') import tensorflow as tf import os import os.path as osp from keras import backend as K def freeze_session(session, keep_var_names=None, output_names=None, clear_devices=True): from tensorflow.python.framework.graph_util import convert_variables_to_constants graph = session.graph with graph.as_default(): freeze_var_names = list(set(v.op.name for v in tf.global_variables()).difference(keep_var_names or [])) output_names = output_names or [] output_names += [v.op.name for v in tf.global_variables()] input_graph_def = graph.as_graph_def() if clear_devices: for node in input_graph_def.node: node.device = "" frozen_graph = convert_variables_to_constants(session, input_graph_def, output_names, freeze_var_names) return frozen_graph input_fld = '/data/codebase/Keyword-fenci/brand_recogniton_biLSTM/' weight_file = 'biLSTM_brand_recognize.model' output_graph_name = 'tensor_model_v3.pb' output_fld = input_fld + '/tensorflow_model/' if not os.path.isdir(output_fld): os.mkdir(output_fld) weight_file_path = osp.join(input_fld, weight_file) K.set_learning_phase(0) net_model = load_model(weight_file_path) print('input is :', net_model.input.name) print ('output is:', net_model.output.name) sess = K.get_session() frozen_graph = freeze_session(K.get_session(), output_names=[net_model.output.op.name]) from tensorflow.python.framework import graph_io graph_io.write_graph(frozen_graph, output_fld, output_graph_name, as_text=True) print('saved the constant graph (ready for inference) at: ', osp.join(output_fld, output_graph_name))
然后模型就存成了.pb格式的文件
问题就来了,这样存下来的.pb格式的文件是frozen model
如果通过TensorFlow serving 启用模型的话,会报错:
E tensorflow_serving/core/aspired_versions_manager.cc:358] Servable {name: mnist version: 1} cannot be loaded: Not found: Could not find meta graph def matching supplied tags: { serve }. To inspect available tag-sets in the SavedModel, please use the SavedModel CLI: `saved_model_cli`
因为TensorFlow serving 希望读取的是saved model
于是需要将frozen model 转化为 saved model 格式,解决方案如下:
from tensorflow.python.saved_model import signature_constants from tensorflow.python.saved_model import tag_constants export_dir = '/data/codebase/Keyword-fenci/brand_recogniton_biLSTM/saved_model' graph_pb = '/data/codebase/Keyword-fenci/brand_recogniton_biLSTM/tensorflow_model/tensor_model.pb' builder = tf.saved_model.builder.SavedModelBuilder(export_dir) with tf.gfile.GFile(graph_pb, "rb") as f: graph_def = tf.GraphDef() graph_def.ParseFromString(f.read()) sigs = {} with tf.Session(graph=tf.Graph()) as sess: # name="" is important to ensure we don't get spurious prefixing tf.import_graph_def(graph_def, name="") g = tf.get_default_graph() inp = g.get_tensor_by_name(net_model.input.name) out = g.get_tensor_by_name(net_model.output.name) sigs[signature_constants.DEFAULT_SERVING_SIGNATURE_DEF_KEY] = \ tf.saved_model.signature_def_utils.predict_signature_def( {"in": inp}, {"out": out}) builder.add_meta_graph_and_variables(sess, [tag_constants.SERVING], signature_def_map=sigs) builder.save()
于是保存下来的saved model 文件夹下就有两个文件:
saved_model.pb variables
其中variables 可以为空
于是将.pb 模型导入serving再读取,成功!
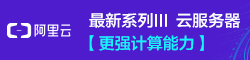
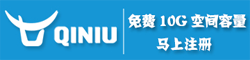