python神经网络Keras实现GRU及其参数量
什么是GRU
GRU是LSTM的一个变种。
传承了LSTM的门结构,但是将LSTM的三个门转化成两个门,分别是更新门和重置门。
1、GRU单元的输入与输出
下图是每个GRU单元的结构。
在n时刻,每个GRU单元的输入有两个:
- 当前时刻网络的输入值Xt;
- 上一时刻GRU的输出值ht-1;
输出有一个:
当前时刻GRU输出值ht;
2、GRU的门结构
GRU含有两个门结构,分别是:
更新门zt和重置门rt:
更新门用于控制前一时刻的状态信息被代入到当前状态的程度,更新门的值越大说明前一时刻的状态信息带入越少,这一时刻的状态信息带入越多。
重置门用于控制忽略前一时刻的状态信息的程度,重置门的值越小说明忽略得越多。
3、GRU的参数量计算
a、更新门
更新门在图中的标号为zt,需要结合ht-1和Xt来决定上一时刻的输出ht-1有多少得到保留,更新门的值越大说明前一时刻的状态信息保留越少,这一时刻的状态信息保留越多。
结合公式我们可以知道:
zt由ht-1和Xt来决定。
当更新门zt的值较大的时候,上一时刻的输出ht-1保留较少,而这一时刻的状态信息保留较多。
b、重置门
重置门在图中的标号为rt,需要结合ht-1和Xt来控制忽略前一时刻的状态信息的程度,重置门的值越小说明忽略得越多。
结合公式我们可以知道:
rt由ht-1和Xt来决定。
当重置门rt的值较小的时候,上一时刻的输出ht-1保留较少,说明忽略得越多。
c、全部参数量
所以所有的门总参数量为:
在Keras中实现GRU
GRU一般需要输入两个参数。
一个是unit、一个是input_shape。
LSTM(CELL_SIZE, input_shape = (TIME_STEPS,INPUT_SIZE))
unit用于指定神经元的数量。
input_shape用于指定输入的shape,分别指定TIME_STEPS和INPUT_SIZE。
实现代码
import numpy as np from keras.models import Sequential from keras.layers import Input,Activation,Dense from keras.models import Model from keras.datasets import mnist from keras.layers.recurrent import GRU from keras.utils import np_utils from keras.optimizers import Adam TIME_STEPS = 28 INPUT_SIZE = 28 BATCH_SIZE = 50 index_start = 0 OUTPUT_SIZE = 10 CELL_SIZE = 75 LR = 1e-3 (X_train,Y_train),(X_test,Y_test) = mnist.load_data() X_train = X_train.reshape(-1,28,28)/255 X_test = X_test.reshape(-1,28,28)/255 Y_train = np_utils.to_categorical(Y_train,num_classes= 10) Y_test = np_utils.to_categorical(Y_test,num_classes= 10) inputs = Input(shape=[TIME_STEPS,INPUT_SIZE]) x = GRU(CELL_SIZE, input_shape = (TIME_STEPS,INPUT_SIZE))(inputs) x = Dense(OUTPUT_SIZE)(x) x = Activation("softmax")(x) model = Model(inputs,x) adam = Adam(LR) model.summary() model.compile(loss = 'categorical_crossentropy',optimizer = adam,metrics = ['accuracy']) for i in range(50000): X_batch = X_train[index_start:index_start + BATCH_SIZE,:,:] Y_batch = Y_train[index_start:index_start + BATCH_SIZE,:] index_start += BATCH_SIZE cost = model.train_on_batch(X_batch,Y_batch) if index_start >= X_train.shape[0]: index_start = 0 if i%100 == 0: cost,accuracy = model.evaluate(X_test,Y_test,batch_size=50) print("accuracy:",accuracy)
实现效果:
10000/10000 [==============================] - 2s 231us/step accuracy: 0.16749999986961484 10000/10000 [==============================] - 2s 206us/step accuracy: 0.6134000015258789 10000/10000 [==============================] - 2s 214us/step accuracy: 0.7058000019192696 10000/10000 [==============================] - 2s 209us/step accuracy: 0.797899999320507
栏 目:Python代码
下一篇:python利用多线程+队列技术爬取中介网互联网网站排行榜
本文标题:python神经网络Keras实现GRU及其参数量
本文地址:http://www.codeinn.net/misctech/225717.html
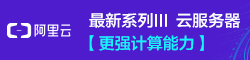
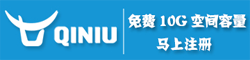