python如何将多个模型的ROC曲线绘制在一张图(含图例)
多条ROC曲线绘制函数
def multi_models_roc(names, sampling_methods, colors, X_test, y_test, save=True, dpin=100): """ 将多个机器模型的roc图输出到一张图上 Args: names: list, 多个模型的名称 sampling_methods: list, 多个模型的实例化对象 save: 选择是否将结果保存(默认为png格式) Returns: 返回图片对象plt """ plt.figure(figsize=(20, 20), dpi=dpin) for (name, method, colorname) in zip(names, sampling_methods, colors): method.fit(X_train, y_train) y_test_preds = method.predict(X_test) y_test_predprob = method.predict_proba(X_test)[:,1] fpr, tpr, thresholds = roc_curve(y_test, y_test_predprob, pos_label=1) plt.plot(fpr, tpr, lw=5, label='{} (AUC={:.3f})'.format(name, auc(fpr, tpr)),color = colorname) plt.plot([0, 1], [0, 1], '--', lw=5, color = 'grey') plt.axis('square') plt.xlim([0, 1]) plt.ylim([0, 1]) plt.xlabel('False Positive Rate',fontsize=20) plt.ylabel('True Positive Rate',fontsize=20) plt.title('ROC Curve',fontsize=25) plt.legend(loc='lower right',fontsize=20) if save: plt.savefig('multi_models_roc.png') return plt
绘制效果
调用格式与方法
调用方法时,需要把模型本身(如clf_xx)、模型名字(如GBDT)和对应颜色(如crimson)按照顺序、以列表形式传入函数作为参数。
names = ['Logistic Regression', 'Random Forest', 'XGBoost', 'AdaBoost', 'GBDT', 'LGBM'] sampling_methods = [clf_lr, clf_rf, clf_xgb, clf_adb, clf_gbdt, clf_lgbm ] colors = ['crimson', 'orange', 'gold', 'mediumseagreen', 'steelblue', 'mediumpurple' ] #ROC curves train_roc_graph = multi_models_roc(names, sampling_methods, colors, X_train, y_train, save = True) train_roc_graph.savefig('ROC_Train_all.png')
详细解释和说明
1.关键函数
(1)plt.figure(figsize=(20, 20), dpi=dpin)
在for循环外绘制图片的大体框架。figsize控制图片大小,dpin控制图片的信息量(其实可以理解为清晰度?documentation的说明是The resolution of the figure in dots-per-inch)
(2)zip()
函数用于将可迭代的对象作为参数,将对象中对应的元素打包成一个个元组,然后返回由这些元组组成的列表。
(3)roc_curve()
fpr, tpr, thresholds = roc_curve(y_test, y_test_predprob, pos_label=1)
该函数的传入参数为目标特征的真实值y_test和模型的预测值y_test_predprob。需要为pos_label赋值,指明正样本的值。
该函数的返回值 fpr、tpr和thresholds 均为ndarray, 为对应每一个不同的阈值下计算出的不同的真阳性率和假阳性率。这些值,就对应着ROC图中的各个点。
(4)auc()
plt.plot(fpr, tpr, lw=5, label='{} (AUC={:.3f})'.format(name, auc(fpr, tpr)),color = colorname)
函数auc(),传入参数为fpr和tpr,返回结果为模型auc值,即曲线下面积值。
以上代码在使用fpr和tpr绘制ROC曲线的同时,也确定了标签(图例)的内容和格式。
2. 参数解释
(1)sampling_methods
是包含多个模型名字的list。所有模型不需要fit过再传入函数,只需要定义好即可。
clf = RandomForestClassifier(n_estimators = 100, max_depth=3, min_samples_split=0.2, random_state=0)
(2)X_test, y_test
X_test 和 y_test 两个参数用于传入函数后计算各个模型的预测值。
y_test_predprob = method.predict_proba(X_test)[:,1] fpr, tpr, thresholds = roc_curve(y_test, y_test_predprob, pos_label=1)
如果需要绘制的是训练集的ROC曲线,则可以在对应参数位置分别传入X_trian和y_train即可。
(3)names 和 colors
这两个参数均为字符串列表形式。注意,这两个列表的值要和模型参数中的模型顺序一一对应。
如有需要绘制更多的模型,只需要对应增加列表中的值即可。
需要注意的小小坑
1.同一张图片的同一种方法只能调用一次!!!
plt.legend(loc='lower right') plt.legend(fontsize=10)
如果像上图中的我一样,把同一张图片plt的方法legend()调用两次,那么下一个的方法中的参数就会将上一个的参数覆盖!这种情况下,我就发现第一个方法赋值的location完全不起作用……
这个时候就需要将这个函数整合如下图~(其实本来就是应该这么写的,我也不知道为啥我脑子一抽写了两个,可能是ggplot给我的美好印象挥之不去吧)
plt.legend(loc='lower right',fontsize=10)
补充
根据小伙伴的评论提问,在这里进行一下解释说明:
1.这个函数是适用于所有数据集的,只需要导入数据集后进行训练集和测试集的划分即可。(我在“调用格式与方法”部分调用函数使用的是X_train 和y_train,绘制出的则是不同模型在训练集表现的ROC曲线)
划分训练集和测试集的代码如下(以使用8:2划分训练集测试集为例)
# 8:2划分训练集测试集 X, y = df.drop(target,axis=1), df[target] X_train, X_test, y_train, y_test = train_test_split(X, y, train_size=0.8, random_state=0)
df:导入数据集
target:目标特征(y)
train_size:训练集占比80%
random_state: 随机数种子,不同随机数种子划分的训练集和测试集会有不同。
总结
上一篇:最炫Python烟花代码全解析
栏 目:Python代码
本文标题:python如何将多个模型的ROC曲线绘制在一张图(含图例)
本文地址:http://www.codeinn.net/misctech/216066.html
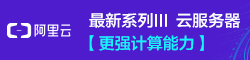
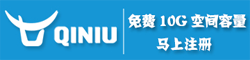