python目标检测SSD算法预测部分源码详解
学习前言
……学习了很多有关目标检测的概念呀,咕噜咕噜,可是要怎么才能进行预测呢,我看了好久的SSD源码,将其中的预测部分提取了出来,训练部分我还没看懂
什么是SSD算法
SSD是一种非常优秀的one-stage方法,one-stage算法就是目标检测和分类是同时完成的,其主要思路是均匀地在图片的不同位置进行密集抽样,抽样时可以采用不同尺度和长宽比,然后利用CNN提取特征后直接进行分类与回归,整个过程只需要一步,所以其优势是速度快。
但是均匀的密集采样的一个重要缺点是训练比较困难,这主要是因为正样本与负样本(背景)极其不均衡(参见Focal Loss),导致模型准确度稍低。
SSD的英文全名是Single Shot MultiBox Detector,Single shot说明SSD算法属于one-stage方法,MultiBox说明SSD算法基于多框预测。(它真的不是固态硬盘啊~~~~~~)
讲解构架
本次教程的讲解分为俩个部分,第一部分是ssd_vgg_300主体的源码的讲解,第二部分是如何调用ssd_vgg_300主体的源码,即利用源码进行预测。
ssd_vgg_300主体的源码的讲解包括如下三个部分:
1、网络部分,用于建立ssd网络,用于预测种类和框的位置。
2、先验框部分,根据每个特征层的shape,构建出合适比例的框,同时可以减少运算量。
3、解码部分,根据网络部分和先验框部分的输出,对框的位置进行解码。
利用源码进行预测的讲解包括以下三个部分:
1、如何对图片进行处理。
2、载入模型
3、预测过程中处理的流程。
在看本次算法前,建议先下载我简化过的源码,配合观看,在其中运行demo即可执行程序:
下载链接:https://pan.baidu.com/s/16UtXIfE-imrzjg_rx7xTKQ
提取码:vpo2
ssd_vgg_300主体的源码
本文使用的ssd_vgg_300的源码源于
链接:https://pan.baidu.com/s/1Wi1t9bYpTJEu5j3cq3pUnA
提取码:6hye
本文对其进行了简化,只保留了预测部分,便于理顺整个SSD的框架。
1、 大体框架
在只需要预测的情况下,需要保留ssd_vgg_300源码的网络部分、先验框部分和解码部分。(这里只能使用图片哈,因为VScode收缩后也不能只复制各个部分的函数名)
其中:
1、net函数用于构建网络,其输入值为shape为(None,300,300,3)的图像,在其中会经过许多层网络结构,在这许多的网络结构中存在6个特征层,用于读取框框,最终输出predictions和locations,predictions和locations中包含6个层的预测结果和框的位置。
2、arg_scope用于初始化网络每一个层的默认参数,该项目会用到slim框架,slim框架是一个轻量级的tensorflow框架,其参数初始化与slim中的函数相关。
3、anchors用于获得先验框,先验框也是针对6个特征层的。
4、bboxes_decode用于结合先验框和locations获得在img中框的位置,locations相当于编码过后的框的位置,这样做可以方便SSD网络学习,bboxes_decode用于解码,解码后可以获得img中框的位置。
2、net网络构建
# =============================网络部分============================= # def net(self, inputs, is_training=True, update_feat_shapes=True, dropout_keep_prob=0.5, prediction_fn=slim.softmax, reuse=None, scope='ssd_300_vgg'): """ SSD 网络定义,调用外部函数,建立网络层 """ r = ssd_net(inputs, num_classes=self.params.num_classes, feat_layers=self.params.feat_layers, anchor_sizes=self.params.anchor_sizes, anchor_ratios=self.params.anchor_ratios, normalizations=self.params.normalizations, is_training=is_training, dropout_keep_prob=dropout_keep_prob, prediction_fn=prediction_fn, reuse=reuse, scope=scope) return r
在net函数中,其调用了一个外部的函数ssd_net,我估计作者是为了让代码主体更简洁。
实际的构建代码在ssd_net函数中,网络构建代码中使用了许多的slim.repeat,该函数用于重复构建卷积层,具体构建的层共11层,在进行目标检测框的选择时,我们选择其中的[‘block4’, ‘block7’, ‘block8’, ‘block9’, ‘block10’, ‘block11’]。
这里我们放出论文中的网络结构层。
通过该图我们可以发现,其网络结构如下:
1、首先通过了多个3X3卷积层、5次步长为2的最大池化取出特征,形成了5个Block,其中第四个Block的shape为(?,38,38,512),该层用于提取小目标(多次卷积后大目标的特征保存的更好,小目标特征会消失,需要在比较靠前的层提取小目标特征)。
2、进行一次卷积核膨胀dilate(关于卷积核膨胀的概念可以去网上搜索以下哈)。
3、读取第七个Block7的特征,shape为(?,19,19,1024)
4、分别利用1x1和3x3卷积提取特征,在3x3卷积的时候使用步长2,缩小特征数。获取第八个Block8的特征,shape为(?,10,10,512)
5、重复步骤4,获得9、10、11卷积层的特征,shape分别为(?,5,5,256)、(?,3,3,256)、(?,1,1,256)
此时网络便构建完了。
# =============================网络部分============================= # ############################################################ # 该部分供SSDNet的net函数调用,用于建立网络 # # 返回predictions, localisations, logits, end_points # ############################################################ def ssd_net(inputs, num_classes=SSDNet.default_params.num_classes, feat_layers=SSDNet.default_params.feat_layers, anchor_sizes=SSDNet.default_params.anchor_sizes, anchor_ratios=SSDNet.default_params.anchor_ratios, normalizations=SSDNet.default_params.normalizations, is_training=True, dropout_keep_prob=0.5, prediction_fn=slim.softmax, reuse=None, scope='ssd_300_vgg'): """SSD net definition. """ # 建立网络 end_points = {} with tf.variable_scope(scope, 'ssd_300_vgg', [inputs], reuse=reuse): # Block1 ''' 相当于执行: net = self.conv2d(x,64,[3,3],scope = 'conv1_1') net = self.conv2d(net,64,[3,3],scope = 'conv1_2') ''' # (300,300,3) -> (300,300,64) -> (150,150,64) net = slim.repeat(inputs, 2, slim.conv2d, 64, [3, 3], scope='conv1') end_points['block1'] = net net = slim.max_pool2d(net, [2, 2], scope='pool1') # Block 2. ''' 相当于执行: net = self.conv2d(net,128,[3,3],scope = 'conv2_1') net = self.conv2d(net,128,[3,3],scope = 'conv2_2') ''' # (150,150,64) -> (150,150,128) -> (75,75,128) net = slim.repeat(net, 2, slim.conv2d, 128, [3, 3], scope='conv2') end_points['block2'] = net net = slim.max_pool2d(net, [2, 2], scope='pool2') # Block 3. ''' 相当于执行: net = self.conv2d(net,256,[3,3],scope = 'conv3_1') net = self.conv2d(net,256,[3,3],scope = 'conv3_2') net = self.conv2d(net,256,[3,3],scope = 'conv3_3') ''' # (75,75,128) -> (75,75,256) -> (38,38,256) net = slim.repeat(net, 3, slim.conv2d, 256, [3, 3], scope='conv3') end_points['block3'] = net net = slim.max_pool2d(net, [2, 2],stride = 2,padding = "SAME", scope='pool3') # Block 4. # 三次卷积 # (38,38,256) -> (38,38,512) -> block4_net -> (19,19,512) net = slim.repeat(net, 3, slim.conv2d, 512, [3, 3], scope='conv4') end_points['block4'] = net net = slim.max_pool2d(net, [2, 2],padding = "SAME", scope='pool4') # Block 5. # 三次卷积 # (19,19,512)->(19,19,512) net = slim.repeat(net, 3, slim.conv2d, 512, [3, 3], scope='conv5') end_points['block5'] = net net = slim.max_pool2d(net, [3, 3], stride=1,padding = "SAME", scope='pool5') # Block 6: dilate # 卷积核膨胀 # (19,19,512)->(19,19,1024) net = slim.conv2d(net, 1024, [3, 3], rate=6, scope='conv6') end_points['block6'] = net net = tf.layers.dropout(net, rate=dropout_keep_prob, training=is_training) # Block 7: 1x1 conv # (19,19,1024)->(19,19,1024) net = slim.conv2d(net, 1024, [1, 1], scope='conv7') end_points['block7'] = net net = tf.layers.dropout(net, rate=dropout_keep_prob, training=is_training) # Block 8/9/10/11: 1x1 and 3x3 convolutions stride 2 (except lasts). # (19,19,1024)->(19,19,256)->(10,10,512) end_point = 'block8' with tf.variable_scope(end_point): net = slim.conv2d(net, 256, [1, 1], scope='conv1x1') net = custom_layers.pad2d(net, pad=(1, 1)) net = slim.conv2d(net, 512, [3, 3], stride=2, scope='conv3x3', padding='VALID') end_points[end_point] = net end_point = 'block9' # (10,10,512)->(10,10,128)->(5,5,256) with tf.variable_scope(end_point): net = slim.conv2d(net, 128, [1, 1], scope='conv1x1') net = custom_layers.pad2d(net, pad=(1, 1)) net = slim.conv2d(net, 256, [3, 3], stride=2, scope='conv3x3', padding='VALID') end_points[end_point] = net end_point = 'block10' # (5,5,256)->(5,5,128)->(3,3,256) with tf.variable_scope(end_point): net = slim.conv2d(net, 128, [1, 1], scope='conv1x1') net = slim.conv2d(net, 256, [3, 3], scope='conv3x3', padding='VALID') end_points[end_point] = net end_point = 'block11' # (3,3,256)->(1,1,256) with tf.variable_scope(end_point): net = slim.conv2d(net, 128, [1, 1], scope='conv1x1') net = slim.conv2d(net, 256, [3, 3], scope='conv3x3', padding='VALID') end_points[end_point] = net # 预测和定位层 predictions = [] logits = [] localisations = [] for i, layer in enumerate(feat_layers): with tf.variable_scope(layer + '_box'): p, l = ssd_multibox_layer(end_points[layer], num_classes, anchor_sizes[i], anchor_ratios[i], normalizations[i]) predictions.append(prediction_fn(p)) logits.append(p) localisations.append(l) return predictions, localisations, logits, end_points ssd_net.default_image_size = 300
仔细看代码的同学会发现,除去层的构建外,最后还多了一段循环,那这个循环是做什么的呢?而且同学们可以感受到,虽然我们提取了特征层,但是这个特征层和预测值、框的位置又有什么关系呢?
这个循环就是用来将特征层转化成预测值和框的位置的。
在循环中我们调用了ssd_multibox_layer函数,该函数的作用如下:
1、读取网络的特征层
2、对网络的特征层再次进行卷积,该卷积分为两部分,互不相干,分别用于预测种类和框的位置。
3、预测框的位置,以Block4为例,Block4的shape为(?,38,38,512),再次卷积后,使其shape变为(?,38,38,num_anchors x 4),其中num_anchors是每个特征点中先验框的数量,4代表框的特点,一个框需要4个特征才可以确定位置,最后再reshape为(?,38,38,num_anchors,4),代表38x38个特点中,第num_anchors个框下的4个特点。
4、预测种类,以Block4为例,Block4的shape为(?,38,38,512),再次卷积后,使其shape变为(?,38,38,num_anchors x 21),其中num_anchors是每个特征点中先验框的数量,21代表预测的种类,包含背景,SSD算法共预测21个种类,最后再reshape为(?,38,38,num_anchors,21),代表38x38个特点中,第num_anchors个框下的21个预测结果。
该函数的输出结果中:
location_pred的shape为(?,feat_block.shape[0],feat_block.shape[1], num_anchors,4)
class_pred的shape为(?,feat_block.shape[0],feat_block.shape[1],num_anchors,21)
具体执行代码如下:
############################################################ # 该部分供ssd_net函数调用,返回种类预测和位置预测 # # 将特征层的内容输入,根据特征层返回预测结果 # ############################################################ def ssd_multibox_layer(inputs, num_classes, sizes, ratios=[1], normalization=-1, bn_normalization=False): reshape = [-1] + inputs.get_shape().as_list()[1:-1] # 去除第一个和最后一个得到shape net = inputs # 对第一个特征层进行l2标准化。 if normalization > 0: net = custom_layers.l2_normalization(net, scaling=True) # Number of anchors. num_anchors = len(sizes) + len(ratios) # Location. num_loc_pred = num_anchors * 4 loc_pred = slim.conv2d(net, num_loc_pred, [3, 3], activation_fn=None, scope='conv_loc') loc_pred = custom_layers.channel_to_last(loc_pred) loc_pred = tf.reshape(loc_pred, reshape + [num_anchors, 4]) # Class prediction. num_cls_pred = num_anchors * num_classes cls_pred = slim.conv2d(net, num_cls_pred, [3, 3], activation_fn=None, scope='conv_cls') cls_pred = custom_layers.channel_to_last(cls_pred) cls_pred = tf.reshape(cls_pred, reshape + [num_anchors, num_classes]) return cls_pred, loc_pred
3、anchor先验框生成
# ==========================生成先验框部分========================== # def anchors(self, img_shape, dtype=np.float32): """ 计算给定图像形状的默认定位框,调用外部函数,获得先验框。 """ return ssd_anchors_all_layers(img_shape, self.params.feat_shapes, self.params.anchor_sizes, self.params.anchor_ratios, self.params.anchor_steps, self.params.anchor_offset, dtype)
在anchor函数中,其调用了一个外部的函数ssd_anchors_all_layers,用于构建先验框。先验框的构建和上述网络的构建关系不大,但是需要用到上述网络net的特征层size,先验框的构建目的是为了让图片构建出合适比例的框,同时可以减少运算量。
在进入ssd_anchors_all_layers函数后,根据名字可以知道,该函数用于生成所有层的先验框,其会进入一个循环,该循环用于根据每个特征层的size进行先验框的构建
代码如下:
############################################################ # 该部分供SSDNet的anchors函数调用,用于获取先验框 # # 返回y,x,h,w的组和 # ############################################################ def ssd_anchors_all_layers(img_shape, layers_shape, anchor_sizes, anchor_ratios, anchor_steps, offset=0.5, dtype=np.float32): """ 对所有特征层进行计算 """ layers_anchors = [] for i, s in enumerate(layers_shape): anchor_bboxes = ssd_anchor_one_layer(img_shape, s, anchor_sizes[i], anchor_ratios[i], anchor_steps[i], offset=offset, dtype=dtype) layers_anchors.append(anchor_bboxes) return layers_anchors
此时再调用ssd_anchor_one_layer,根据名字可以知道,该函数用于生成单层的先验框,该部分是先验框生成的核心。
输入参数包括图像大小img_shape,特征层大小feat_shape,先验框大小sizes,先验框长宽比率sizes,先验框放大倍数step。
执行过程:
1、根据feat_shape生成x、y的网格。
2、将x和y归一化到0到1之间,这里的x和y对应每个特征层的每一个点,同时x,y对应每个框的中心。
3、生成每个特征层的每个点对应的num_anchors大小相同的h和w,即4、6、6、6、4、4,这里的h和w对应着每一个点对应的num_anchors个框中的h和w。
4、将h和w每个赋值,h[0]对应比较小的正方形,h[1]对应比较大的正方形,h[2]和h[3]对应√2下不同的长方形,h[4]和h[5]对应√3下不同的长方形。
输出的参数包括:
X和Y的shape为(block.shape[0],block.shape[1],1)
H和w的shape为(boxes_len)
具体的执行代码如下:
############################################################ # 该部分供ssd_anchors_all_layers函数调用 # # 用于获取单层的先验框返回y,x,h,w # ############################################################ def ssd_anchor_one_layer(img_shape, feat_shape, sizes, ratios, step, offset=0.5, dtype=np.float32): """ 输入:图像大小img_shape,特征层大小feat_shape,先验框大小sizes, 先验框长宽比率sizes,先验框放大倍数step。 执行过程: 生成x、y的网格。 将x和y归一化到0到1之间。 生成每个特征层的每个点对应的boxes_len大小相同的h和w,即4、6、6、6、4、4。 将h和w每个赋值,h[0]对应比较小的正方形,h[1]对应比较大的正方形, h[2]和h[3]对应√2下不同的长方形,h[4]和h[5]对应√3下不同的长方形。 输出: X和Y的shape为(block.shape[0],block.shape[1],1) H和w的shape为(boxes_len) """ # 网格化 y, x = np.mgrid[0:feat_shape[0], 0:feat_shape[1]] # 归一化 y = (y.astype(dtype) + offset) * step / img_shape[0] x = (x.astype(dtype) + offset) * step / img_shape[1] # 拓充维度,便于后面decode计算 y = np.expand_dims(y, axis=-1) x = np.expand_dims(x, axis=-1) # 每一个点框框的数量 num_anchors = len(sizes) + len(ratios) h = np.zeros((num_anchors, ), dtype=dtype) w = np.zeros((num_anchors, ), dtype=dtype) # 第一个第二个框框是正方形 h[0] = sizes[0] / img_shape[0] w[0] = sizes[0] / img_shape[1] di = 1 if len(sizes) > 1: h[1] = math.sqrt(sizes[0] * sizes[1]) / img_shape[0] w[1] = math.sqrt(sizes[0] * sizes[1]) / img_shape[1] di += 1 for i, r in enumerate(ratios): h[i+di] = sizes[0] / img_shape[0] / math.sqrt(r) w[i+di] = sizes[0] / img_shape[1] * math.sqrt(r) return y, x, h, w
在看该部分的时候,需要结合参数,所用参数如下:
img_shape=(300, 300) feat_shapes=[(38, 38), (19, 19), (10, 10), (5, 5), (3, 3), (1, 1)], # 先验框的size anchor_sizes=[(21., 45.), (45., 99.), (99., 153.), (153., 207.), (207., 261.), (261., 315.)], # 框的数量为4,6,6,6,4,4 # 框的数量为2+len(anchor_ratios[i]) anchor_ratios=[[2, .5], [2, .5, 3, 1./3], [2, .5, 3, 1./3], [2, .5, 3, 1./3], [2, .5], [2, .5]], # 放大倍数 anchor_steps=[8, 16, 32, 64, 100, 300],
仔细研读这段代码会发现其设计非常巧妙哈。
x和y会执行归一化,到0,1之间,如果除去xy的最后一维进行plot,其会呈现一个0到1的网格,以38x38的特征层的先验框为例,其绘制出的网格如下,其实每一个点对应的就是每个框的中心点。
h和w对应着每个框的高与宽,宽高成一定比例。
4、bboxes_decode框的解码
# =============================解码部分============================= # def bboxes_decode(self, feat_localizations, anchors, scope='ssd_bboxes_decode'): """ 进行解码操作 """ return ssd_common.tf_ssd_bboxes_decode( feat_localizations, anchors, prior_scaling=self.params.prior_scaling, scope=scope)
在bboxes_decode函数中,其调用了一个外部的函数ssd_common.tf_ssd_bboxes_decode,用于构建框的解码,其位于其它的文件中。
执行框的解码的原因是,利用net网络预测得到的locations并不是实际的框的位置,其需要与先验框结合处理后才能得到最后的框的位置。
这里需要注意的是,decode的过程需要两个参数的结合,分别是net网络构建得到的locations和anchor先验框生成得到的先验框。
在进入ssd_common.tf_ssd_bboxes_decode函数后,其执行过程与anchor先验框生成类似,内部也有一个循环,意味着要对每一个特征层进行单独的处理。
def tf_ssd_bboxes_decode(feat_localizations, anchors, prior_scaling=[0.1, 0.1, 0.2, 0.2], scope='ssd_bboxes_decode'): """ 从ssd网络特性和先验框框计算相对边界框。 """ with tf.name_scope(scope): bboxes = [] for i, anchors_layer in enumerate(anchors): bboxes.append( tf_ssd_bboxes_decode_layer(feat_localizations[i], anchors_layer, prior_scaling)) return bboxes
在如上的执行过程中,内部存在一个tf_ssd_bboxes_decode_layer函数,该部分是先验框生成的核心,在tf_ssd_bboxes_decode_layer中,程序会对每一个特征层的框进行解码。
其输入包括,一个特征层的框的预测定位feat_localizations,每一层的先验框anchors_layer,先验框比率prior_scaling
执行过程:
1、 分解anchors_layer,因为anchors_layer由多个y,x,h,w构成
2、 计算cx和cy,这里存在一个计算公式,公式论文中给出了。
3、 计算cw和ch,这里存在一个计算公式,公式论文中给出了。
4、 将[cy - ch / 2.0, cx - cw / 2.0, cy + ch / 2.0, cx + cw / 2.0]输出,其对应左上角角点和右下角角点。
其输出包括:左上角角点和右下角角点的集合bboxes。
bboxes的shape为(?,block.shape[0],block.shape[1], boxes_len,4)
具体执行代码如下:
# =========================================================================== # # 编码解码部分 # =========================================================================== # def tf_ssd_bboxes_decode_layer(feat_localizations, anchors_layer, prior_scaling=[0.1, 0.1, 0.2, 0.2]): """ 其输入包括,一个特征层的框的预测定位feat_localizations,每一层的先验框anchors_layer,先验框比率prior_scaling 执行过程: 1、 分解anchors_layer,因为anchors_layer由多个y,x,h,w构成 2、 计算cx和cy,这里存在一个计算公式 3、 计算cw和ch,这里存在一个计算公式 4、 将[cy - ch / 2.0, cx - cw / 2.0, cy + ch / 2.0, cx + cw / 2.0]输出,其对应左上角角点和右下角角点。 其输出包括:左上角角点和右下角角点的集合bboxes。 bboxes的shape为(?,block.shape[0],block.shape[1], boxes_len,4) """ yref, xref, href, wref = anchors_layer # 计算中心点和它的宽长 cx = feat_localizations[:, :, :, :, 0] * wref * prior_scaling[0] + xref cy = feat_localizations[:, :, :, :, 1] * href * prior_scaling[1] + yref w = wref * tf.exp(feat_localizations[:, :, :, :, 2] * prior_scaling[2]) h = href * tf.exp(feat_localizations[:, :, :, :, 3] * prior_scaling[3]) # 计算左上角点和右下角点 ymin = cy - h / 2. xmin = cx - w / 2. ymax = cy + h / 2. xmax = cx + w / 2. bboxes = tf.stack([ymin, xmin, ymax, xmax], axis=-1) return bboxes
解码完后的bboxes表示某一个特征层中的框在真实图像中的位置。
利用ssd_vgg_300进行预测
预测步骤
进行预测需要进行以下步骤:
1、建立ssd对象
2、利用ssd_net = ssd_vgg_300.SSDNet()获得网络,得到两个tensorflow格式的预测结果。
3、载入ssd模型。
4、读入图片image_names。
5、将图片预处理后,传入网络结构,获得预测结果,预测结果包括 框的位置、每个框的预测结果。
6、利用ssd_bboxes_select函数选择得分高于门限的框。
7、对所有的得分进行排序,取出得分top400的框
8、非极大值抑制,该部分用于去除重复率过高的框。
9、在原图中绘制框框。
具体预测过程
1、图片的预处理
图片预处理时,需要调用如下代码:
# 输入图片大小 net_shape = (300, 300) # data_format 设置为 "NHWC" 时,排列顺序为 [batch, height, width, channels] # 具体使用方法可以查看该网址:https://www.jianshu.com/p/d8a699745529 data_format = 'NHWC' # img_input的placeholder img_input = tf.placeholder(tf.uint8, shape = (None, None, 3)) # 对图片进行预处理,得到bbox_img和image_4d image_pre, labels_pre, bboxes_pre, bbox_img = ssd_vgg_preprocessing.preprocess_for_eval( img_input, None, None, net_shape, data_format, resize = ssd_vgg_preprocessing.Resize.WARP_RESIZE) # 由于只检测一张图片,所以需要在第一维添加一维度 image_4d = tf.expand_dims(image_pre, 0)
看起来代码很长,特别是倒数第二段代码,但是其实里面什么也没有。
ssd_vgg_preprocessing.preprocess_for_eval的主要执行过程就是:
1、将image减去voc2012得到的所有图片的RGB平均值。
2、增加比例预处理框(这个的作用我不太懂,我觉得它的意思应该就是这个图片可能是一个大图片里面截出的一小个图片,需要对这个比例进行缩放,但是实际运用的时候应该就是一个大图片)。
3、将图片resize到300x300。
4、判断使用CPU还是GPU。
def preprocess_for_eval(image, labels, bboxes, out_shape=EVAL_SIZE, data_format='NHWC', difficults=None, resize=Resize.WARP_RESIZE, scope='ssd_preprocessing_train'): """ 预处理 """ with tf.name_scope(scope): if image.get_shape().ndims != 3: raise ValueError('Input must be of size [height, width, C>0]') # 将image减去voc2012得到的所有图片的RGB平均值 image = tf.to_float(image) image = tf_image_whitened(image, [_R_MEAN, _G_MEAN, _B_MEAN]) # 增加比例预处理框 bbox_img = tf.constant([[0., 0., 1., 1.]]) if bboxes is None: bboxes = bbox_img else: bboxes = tf.concat([bbox_img, bboxes], axis=0) # 这一大段其实只调用了最后一个elif # 将图片resize到300x300 if resize == Resize.NONE: # No resizing... pass elif resize == Resize.CENTRAL_CROP: # Central cropping of the image. image, bboxes = tf_image.resize_image_bboxes_with_crop_or_pad( image, bboxes, out_shape[0], out_shape[1]) elif resize == Resize.PAD_AND_RESIZE: # Resize image first: find the correct factor... shape = tf.shape(image) factor = tf.minimum(tf.to_double(1.0), tf.minimum(tf.to_double(out_shape[0] / shape[0]), tf.to_double(out_shape[1] / shape[1]))) resize_shape = factor * tf.to_double(shape[0:2]) resize_shape = tf.cast(tf.floor(resize_shape), tf.int32) image = tf_image.resize_image(image, resize_shape, method=tf.image.ResizeMethod.BILINEAR, align_corners=False) # Pad to expected size. image, bboxes = tf_image.resize_image_bboxes_with_crop_or_pad( image, bboxes, out_shape[0], out_shape[1]) elif resize == Resize.WARP_RESIZE: # Warp resize of the image. image = tf_image.resize_image(image, out_shape, method=tf.image.ResizeMethod.BILINEAR, align_corners=False) # 分割比例box bbox_img = bboxes[0] bboxes = bboxes[1:] # ……不知道干嘛 if difficults is not None: mask = tf.logical_not(tf.cast(difficults, tf.bool)) labels = tf.boolean_mask(labels, mask) bboxes = tf.boolean_mask(bboxes, mask) # 看使用cpu还是GPU if data_format == 'NCHW': image = tf.transpose(image, perm=(2, 0, 1)) return image, labels, bboxes, bbox_img
2、载入ssd模型
载入ssd模型分为以下几步:
1、建立Session会话
2、建立ssd网络
3、载入模型
执行代码如下:
# 载入ssd的模型 # 建立Session() isess = tf.Session() reuse = True if 'ssd_net' in locals() else None # 建立网络 ssd_net = ssd_vgg_300.SSDNet() with slim.arg_scope(ssd_net.arg_scope(data_format = data_format)): predictions, localisations, _, _ = ssd_net.net(image_4d, is_training = False, reuse = reuse) # 载入模型 ckpt_filename = 'D:/Collection/SSD-Tensorflow-master/logs/model.ckpt-18602' isess.run(tf.global_variables_initializer()) saver = tf.train.Saver() saver.restore(isess, ckpt_filename)
3、读取图片进行预测
该部分需要进行如下操作:
1、获取先验框。
2、读取图片。
3、将图片放入已经完成载入的模型,得到predictions和locations。
4、将每个特征层的预测结果都进行筛选,得分小于threshold的都剔除,并使得所有特征层的预测结果都并排存入一个list。
5、对所有的预测结果进行得分的排序,取出top400的框框。
6、进行非极大抑制,取出重复率过高的框。
7、在原图中绘制框。
具体执行代码如下:
# 获得所有先验框,六个特征层的 ssd_anchors = ssd_net.anchors(net_shape) def process_image(img, select_threshold = 0.5, nms_threshold = .45, net_shape = (300, 300)): # 运行SSD模型 rimg, rpredictions, rlocalisations, rbbox_img = isess.run([image_4d, predictions, localisations, bbox_img], feed_dict = {img_input: img}) # 得到20个类的得分,框框的位置 rclasses, rscores, rbboxes = np_methods.ssd_bboxes_select( rpredictions, rlocalisations, ssd_anchors, select_threshold = select_threshold, img_shape = net_shape, num_classes = 21, decode = True) # 防止超出边界 rbboxes = np_methods.bboxes_clip(rbbox_img, rbboxes) # 取出top400,并通过极大值抑制除去类似框 rclasses, rscores, rbboxes = np_methods.bboxes_sort(rclasses, rscores, rbboxes, top_k = 400) rclasses, rscores, rbboxes = np_methods.bboxes_nms(rclasses, rscores, rbboxes, nms_threshold = nms_threshold) # 在img里进行等比例缩放resize rbboxes = np_methods.bboxes_resize(rbbox_img, rbboxes) return rclasses, rscores, rbboxes # 读取图片 img = mpimg.imread('./street.jpg') # 进行图片的预测 rclasses, rscores, rbboxes = process_image(img) visualization.plt_bboxes(img, rclasses, rscores, rbboxes)
其中,预测结果筛选的代码如下:
- 该部分首先解码;
- 再将每个特征层进行reshape完成平铺;
- 读出除去背景的得分;
- 将得分多余threshold的类进行保存,小于的进行剔除;
- 利用np.concatenate将结果同一排摆放。
def ssd_bboxes_select_layer(predictions_layer, localizations_layer, anchors_layer, select_threshold=0.5, img_shape=(300, 300), num_classes=21, decode=True): """ 选择大于门限的框 """ # 对框进行解码 if decode: localizations_layer = ssd_bboxes_decode(localizations_layer, anchors_layer) # 将所有预测结果变为3维,第一维度维batch,第二维度为size,第三维度为class_num | 4 p_shape = predictions_layer.shape batch_size = p_shape[0] if len(p_shape) == 5 else 1 predictions_layer = np.reshape(predictions_layer, (batch_size, -1, p_shape[-1])) l_shape = localizations_layer.shape localizations_layer = np.reshape(localizations_layer, (batch_size, -1, l_shape[-1])) if select_threshold is None or select_threshold == 0: classes = np.argmax(predictions_layer, axis=2) scores = np.amax(predictions_layer, axis=2) mask = (classes > 0) classes = classes[mask] scores = scores[mask] bboxes = localizations_layer[mask] else: # 取出所有的预测结果 sub_predictions = predictions_layer[:, :, 1:] # 判断哪里的预测结果大于门限 idxes = np.where(sub_predictions > select_threshold) # 如果大于门限则留下,并+1,除去背景 classes = idxes[-1]+1 # 取出所有分数 scores = sub_predictions[idxes] # 和框的位置 bboxes = localizations_layer[idxes[:-1]] return classes, scores, bboxes
对所有的预测结果进行得分的排序,取出top400的框框的过程非常简单,代码如下:
首先利用argsort对得分进行排序,并从大到小排序得分的序号;
取出种类classes、得分scores、框bboxes的top400个。
def bboxes_sort(classes, scores, bboxes, top_k=400): """ 进行排序筛选 """ idxes = np.argsort(-scores) classes = classes[idxes][:top_k] scores = scores[idxes][:top_k] bboxes = bboxes[idxes][:top_k] return classes, scores, bboxes
进行非极大抑制的过程也比较简单,具体代码如下:
将bboxes中每一个框,从得分最高到得分最低依次与其之后所有的框比较;
IOU较小或者属于不同类的框得到保留;
def bboxes_nms(classes, scores, bboxes, nms_threshold=0.45): """ 非极大抑制,去除重复率过大的框. """ keep_bboxes = np.ones(scores.shape, dtype=np.bool) for i in range(scores.size-1): if keep_bboxes[i]: # 计算重叠区域 overlap = bboxes_jaccard(bboxes[i], bboxes[(i+1):]) # 保留重叠区域不是很大或者种类不同的 keep_overlap = np.logical_or(overlap < nms_threshold, classes[(i+1):] != classes[i]) keep_bboxes[(i+1):] = np.logical_and(keep_bboxes[(i+1):], keep_overlap) # 保留重叠部分小或者种类不同的 idxes = np.where(keep_bboxes) return classes[idxes], scores[idxes], bboxes[idxes]
4、全部预测代码
import os import math import random import numpy as np import tensorflow as tf import cv2 import matplotlib.pyplot as plt import matplotlib.image as mpimg import sys sys.path.append('./') from nets import ssd_vgg_300, ssd_common, np_methods from preprocessing import ssd_vgg_preprocessing from notebooks import visualization # 构建slim框架。 slim = tf.contrib.slim # 输入图片大小 net_shape = (300, 300) # data_format 设置为 "NHWC" 时,排列顺序为 [batch, height, width, channels] # 具体使用方法可以查看:https://www.jianshu.com/p/d8a699745529。 data_format = 'NHWC' # img_input的placeholder img_input = tf.placeholder(tf.uint8, shape = (None, None, 3)) # 对图片进行预处理,得到bbox_img和image_4d image_pre, labels_pre, bboxes_pre, bbox_img = ssd_vgg_preprocessing.preprocess_for_eval( img_input, None, None, net_shape, data_format, resize = ssd_vgg_preprocessing.Resize.WARP_RESIZE) # 由于只检测一张图片,所以需要在第一维添加一维度 image_4d = tf.expand_dims(image_pre, 0) # 载入ssd的模型 # 建立Session() isess = tf.Session() reuse = True if 'ssd_net' in locals() else None # 建立网络 ssd_net = ssd_vgg_300.SSDNet() with slim.arg_scope(ssd_net.arg_scope(data_format = data_format)): predictions, localisations, _, _ = ssd_net.net(image_4d, is_training = False, reuse = reuse) # 载入模型 ckpt_filename = './logs/model.ckpt-1498' isess.run(tf.global_variables_initializer()) saver = tf.train.Saver() saver.restore(isess, ckpt_filename) # 获得所有先验框,六个特征层的 ssd_anchors = ssd_net.anchors(net_shape) def process_image(img, select_threshold = 0.5, nms_threshold = .45, net_shape = (300, 300)): # 运行SSD模型 rimg, rpredictions, rlocalisations, rbbox_img = isess.run([image_4d, predictions, localisations, bbox_img], feed_dict = {img_input: img}) # 得到20个类的得分,框框的位置 rclasses, rscores, rbboxes = np_methods.ssd_bboxes_select( rpredictions, rlocalisations, ssd_anchors, select_threshold = select_threshold, img_shape = net_shape, num_classes = 21, decode = True) # 防止超出边界 rbboxes = np_methods.bboxes_clip(rbbox_img, rbboxes) # 取出top400,并通过极大值抑制除去类似框 rclasses, rscores, rbboxes = np_methods.bboxes_sort(rclasses, rscores, rbboxes, top_k = 400) rclasses, rscores, rbboxes = np_methods.bboxes_nms(rclasses, rscores, rbboxes, nms_threshold = nms_threshold) # 在img里进行等比例缩放resize rbboxes = np_methods.bboxes_resize(rbbox_img, rbboxes) return rclasses, rscores, rbboxes # 读取图片 img = mpimg.imread('./street.jpg') # 进行图片的预测 rclasses, rscores, rbboxes = process_image(img) visualization.plt_bboxes(img, rclasses, rscores, rbboxes)
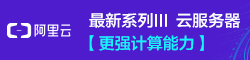
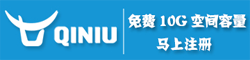