正则化DropPath/drop_path用法示例(Python实现)
DropPath/drop_path 是一种正则化手段,其效果是将深度学习模型中的多分支结构随机”删除“,python中实现如下所示:
def drop_path(x, drop_prob: float = 0., training: bool = False): if drop_prob == 0. or not training: return x keep_prob = 1 - drop_prob shape = (x.shape[0],) + (1,) * (x.ndim - 1) random_tensor = keep_prob + torch.rand(shape, dtype=x.dtype, device=x.device) random_tensor.floor_() # binarize output = x.div(keep_prob) * random_tensor return output class DropPath(nn.Module): def __init__(self, drop_prob=None): super(DropPath, self).__init__() self.drop_prob = drop_prob def forward(self, x): return drop_path(x, self.drop_prob, self.training)
调用如下:
self.drop_path = DropPath(drop_prob) if drop_prob > 0. else nn.Identity() x = x + self.drop_path(self.token_mixer(self.norm1(x))) x = x + self.drop_path(self.mlp(self.norm2(x)))
看起来似乎有点迷茫,这怎么就随机删除了分支呢
实验如下:
import torch drop_prob = 0.2 keep_prob = 1 - drop_prob x = torch.randn(4, 3, 2, 2) shape = (x.shape[0],) + (1,) * (x.ndim - 1) random_tensor = keep_prob + torch.rand(shape, dtype=x.dtype, device=x.device) random_tensor.floor_() output = x.div(keep_prob) * random_tensor
输出:
x.size():[4,3,2,2]
x:
tensor([[[[ 1.3833, -0.3703],
[-0.4608, 0.6955]],
[[ 0.8306, 0.6882],
[ 2.2375, 1.6158]],
[[-0.7108, 1.0498],
[ 0.6783, 1.5673]]],[[[-0.0258, -1.7539],
[-2.0789, -0.9648]],
[[ 0.8598, 0.9351],
[-0.3405, 0.0070]],
[[ 0.3069, -1.5878],
[-1.1333, -0.5932]]],[[[ 1.0379, 0.6277],
[ 0.0153, -0.4764]],
[[ 1.0115, -0.0271],
[ 1.6610, -0.2410]],
[[ 0.0681, -2.0821],
[ 0.6137, 0.1157]]],[[[ 0.5350, -2.8424],
[ 0.6648, -1.6652]],
[[ 0.0122, 0.3389],
[-1.1071, -0.6179]],
[[-0.1843, -1.3026],
[-0.3247, 0.3710]]]])
random_tensor.size():[4, 1, 1, 1] random_tensor: tensor([[[[0.]]], [[[1.]]], [[[1.]]], [[[1.]]]])
output.size():[4,3,2,2] output: tensor([[[[ 0.0000, -0.0000], [-0.0000, 0.0000]], [[ 0.0000, 0.0000], [ 0.0000, 0.0000]], [[-0.0000, 0.0000], [ 0.0000, 0.0000]]], [[[-0.0322, -2.1924], [-2.5986, -1.2060]], [[ 1.0748, 1.1689], [-0.4256, 0.0088]], [[ 0.3836, -1.9848], [-1.4166, -0.7415]]], [[[ 1.2974, 0.7846], [ 0.0192, -0.5955]], [[ 1.2644, -0.0339], [ 2.0762, -0.3012]], [[ 0.0851, -2.6027], [ 0.7671, 0.1446]]], [[[ 0.6687, -3.5530], [ 0.8310, -2.0815]], [[ 0.0152, 0.4236], [-1.3839, -0.7723]], [[-0.2303, -1.6282], [-0.4059, 0.4638]]]])
random_tensor作为是否保留分支的直接置0项,若drop_path的概率设为0.2,random_tensor中的数有0.2的概率为0,而output中被保留概率为0.8。
结合drop_path的调用,若x为输入的张量,其通道为[B,C,H,W],那么drop_path的含义为在一个Batch_size中,随机有drop_prob的样本,不经过主干,而直接由分支进行恒等映射。
总结
栏 目:Python代码
本文标题:正则化DropPath/drop_path用法示例(Python实现)
本文地址:http://www.codeinn.net/misctech/205071.html
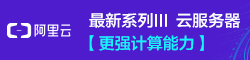
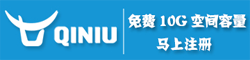